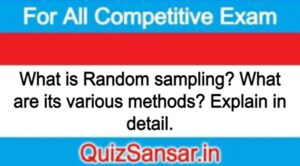
What is Random sampling? What are its various methods? Explain in detail.
What is Random sampling? What are its various methods? Explain in detail.
Or
Describe the various methods of drawing a random sampling from a finite population.
Ans.
Random Sampling
Probability sampling methods are those in which every item in the universe has a known chance or probability of being chosen for the sample. This implies that the selection of sample items is independent of the making the study, that is the sampling operation is controlled so objectively that items will be selected strictly at random.
It may be noted that the term random sample is not used to describe the data in the sample but the process employed to select the sample. Randomness is thus a property of the sampling in a number of ways and hence random example may be of many types.
Random or Probability Sampling
- Simple random sampling
- Stratified sampling
- systematic sampling
- multistage sampling
- cluster sampling
Random of Probability sampling methods
1. Simple Random Sampling
Simple random sampling refers to that sampling technique in which each and very unit of the population has an equal opportunity of being selected in the sample. In simple random sampling, which items get selected in the sample is just a matter of chance. Personal bias of the investigator does not influence the selection. It should be kept in mind that the word ‘random’ does not mean ‘haphazard’ or ‘hit-or-miss’. It means that the selection process is such that chance only determines which items shall be included in the sample. As pointed out by Ya-Lun Chou, when a sample of size n is drawn from a population with N elements, the sample is a ‘simple random sample’ if any of the following is true.
The following are three important elements of sample random sampling methods-
(i) All n items of the sample are selected independently of one another and all N items in the population have the same chance of being included in the sample. By independence of selection, we mean that the selection of a particular item in one draw has no influence on the probabilities of selection in any other draw.
(ii) At each selection, all remaining items in the population have the same equal chance of being selected. If sampling in made with replacement, i.e., when each unit drawn from the universe is replaced before drawing the next unit, each item has a probability of 1/N of being drawn at each selection. If sampling is without replacement, i.e., when each unit drawn from the population is not returned prior to drawing the next unit, the probability of selection of each item remaining in the population at the first draw is 1/N, at the second draw it is 1/(N-1), at the third draw it is 1(N-2) and so on. It should be noted that sampling with replacement has very limited and special use in statistics. We are usually concerned with sampling without replacement.
(iii) All the possible samples of a given size n are equally likely to be selected.
2. Stratified Random Sampling
Simple random sampling is often not convenient or accurate particularly when the population is heterogeneous. There is also a probability of some parts of the population being kept out by chance. Simple random sampling is more commonly used in the case of homogeneous populations.
If a population can be divided into sub-populations or strata the elements in each of which are of a fairly uniform nature, a simple random sample can be drawn from each stratum and the results thus obtained from all the strata are pooled together to obtain a precise estimate for the population. This is stratified sampling which can also be used when specific information from different parts of the population is needed. These parts can be treated as populations in their own right. It is sometimes possible that administratively it may be more convenient to deal with strata rather than a whole population or that a different approach to different strata may be desirable because of non-uniformity.
The size of a stratified sample may be either proportional or non proportional to the stratum size. We may draw, for instance, a 5 per cent or 10 per cent sample from each stratum. A non-proportional sample, however, may be more efficient in many cases.
If the observations in some strata are cheaper to select and to survey or if the observations are highly variable in some strata it may be advisable to take larger samples from such strata.
Estimation of population parameters from non-proportional sample statistics can be done by weighting each subsample statistic in proportion to the size of the corresponding subpopulation.
The chief merits of stratified sampling are low cost, greater accuracy, allowance for geographical concentration and a better coverage.
The method requires a careful division of the population into strata and a truly random sampling in each of these strata.
3. Multistage Sampling
When a survey is to be carried out over a wide area involving heavy travel expenses, use can be made of multistage sampling where :
(i) the population is first divided into large groups or first stage units; one of the first stage units is first selected to be used in the second stage.
(ii) this is divided into smaller, second stage units, and a sample selected out of these; further we may go to third stage units and so on till we arrive at a reasonable sized group to be utilised for the selection of the ultimate sample.
4. Cluster Sampling Or Subsampling
The use of a sampling unit which consists of a group or cluster of the elements in the population is known as cluster sampling. The selection of the sample is done in two stages. First certain groups or clusters are selected from the population. These are primary sampling units. From each of these clusters, elementary sampling units are drawn. From this follow alternative names for this method of sampling viz., subsampling or two stage sampling or block or area sampling. Of course, the process could be continued for multistage sampling.
Cluster sampling permits groups of observations for easier coverage. Travel expenses may be significantly reduced and thus the cost per elementary sampling unit becomes much less.
The results of a cluster sample may not be as precise as those of a random sample but they can be made more precise by taking a larger sample size.
5. Systematic Sampling
Systematic sampling is a particular case of cluster sampling in which the sample is a single cluster. Here a sample is selected at a predecided sampling interval. Thus, every 10th unit in the population gives us a 10 per cent sample. In general every nt unit may be selected. This method has an advantage over random sampling if neighbouring observations resemble one another. The method serves almost the same purpose as stratification in distributing the sampling units more evenly over the entire population.
Systematic sampling being quasi random in nature, may sometimes give misleading results when for instance, every nth unit selected happens to be of the same type. Precaution can help, however. Reliability of information obtained from systematic sampling, in general, is adequate.
The merit of systematic sampling lies in that it is time and money saving if it is handled carefully. A biased sample may mar the utility of the result.
6. Sequential Sampling
Sequential sampling consists in testing a small number of items from a lot of units and accepting and rejecting the whole lot depending on the decision taken with the help of the sample. If no decision can be taken from the first sample, more samples are untaken till a decision is possible. Sequential sampling is used mostly in production units to sample manufactured products.